How can AI models predict drug interactions and side effects with greater accuracy?
How can AI models predict drug interactions and side effects with greater accuracy?
by Maximilian 03:09pm Jan 30, 2025
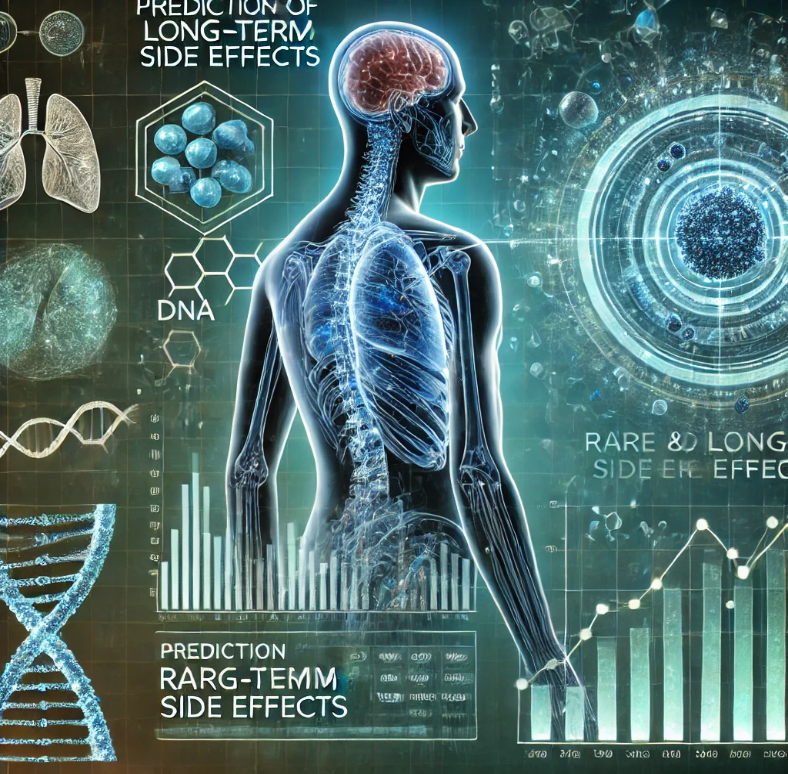
AI models predict drug interactions and side effects with greater accuracy by leveraging advanced computational techniques, large datasets, and integrative approaches. Here are the primary ways AI achieves this:
1. Data Integration and Analysis
AI models combine and analyze diverse data sources to identify patterns and correlations:
Electronic Health Records (EHRs): AI scans patient records to identify real-world drug interaction cases and side effects.
Genomics and Proteomics: AI uses data on genetic mutations, protein structures, and metabolic pathways to understand drug mechanisms.
Chemical Databases: Public databases (e.g., Drug Bank, PubChem) provide information about drug properties, molecular interactions, and known side effects.
Pharmacovigilance Reports: AI processes reports from regulatory bodies and post-market surveillance to detect emerging interaction signals.
2. Predictive Modeling Techniques
Machine Learning (ML) Models:
Supervised Learning: Trained on labeled data about drug interactions and side effects, these models predict outcomes for new combinations.
Unsupervised Learning: Clustering algorithms uncover unknown relationships between drugs based on chemical structure, metabolism, or patient outcomes.
Deep Learning:
Neural networks can process high-dimensional data to identify non-linear relationships, such as rare or complex drug interactions.
Graph neural networks model drug interaction networks, considering drugs, genes, and diseases as interconnected nodes.
Natural Language Processing (NLP):
NLP techniques extract valuable insights from unstructured data, such as clinical notes, research articles, and patient forums.
3. Biological and Mechanistic Modeling
AI simulates biological systems to predict interactions:
Virtual Screening: AI identifies potential molecular targets and interactions by simulating how drugs bind to receptors or enzymes.
Pathway Analysis: Models map drugs to biological pathways to predict cascading effects of interactions.
Pharmacokinetics and Pharmacodynamics (PK/PD): AI predicts how drugs are absorbed, distributed, metabolized, and excreted, and their impact on the body.
4. Real-World Data Utilization
AI enhances prediction accuracy by analyzing real-world patient data:
Adverse Event Reporting Systems: AI correlates reported side effects with patient demographics, co-medications, and comorbidities.
Wearable Devices: Continuous monitoring of vital signs and biomarkers allows AI to detect subtle side effects early.
Population-Level Insights: AI models detect patterns in large-scale data, identifying risks associated with specific drug combinations or populations.
5. Drug Interaction Networks
AI builds comprehensive interaction maps:
Drug-Drug Interaction (DDI) Networks: These visualize relationships between drugs, identifying clusters of high-risk combinations.
Polypharmacy Analysis: AI assesses the cumulative effects of multiple drugs often prescribed together, especially for elderly or chronically ill patients.
6. Predicting Rare and Long-Term Side Effects
Synthetic Data Generation: AI can simulate patient scenarios to predict side effects that are rare or appear after long-term use.
Causal Inference Models: By identifying causal relationships rather than correlations, AI distinguishes true interactions from coincidental occurrences.
Challenges and Innovations
Data Quality: AI performance depends on high-quality, unbiased data. Advances in data cleaning and augmentation improve predictions.
Explain ability: AI models are increasingly using interpretable techniques to provide clinicians with understandable reasoning for predictions.
Validation and Integration: AI predictions require rigorous clinical validation to ensure they are actionable and reliable.
Conclusion
AI models predict drug interactions and side effects with greater accuracy by integrating vast and diverse datasets, leveraging advanced ML/DL techniques, and simulating biological systems. These tools empower healthcare providers and researchers to optimize drug safety, personalize treatments, and reduce adverse outcomes.
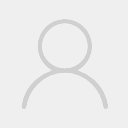