How can machine learning be used to predict and manage market risks?
How can machine learning be used to predict and manage market risks?
by Maximilian 03:24pm Jan 30, 2025
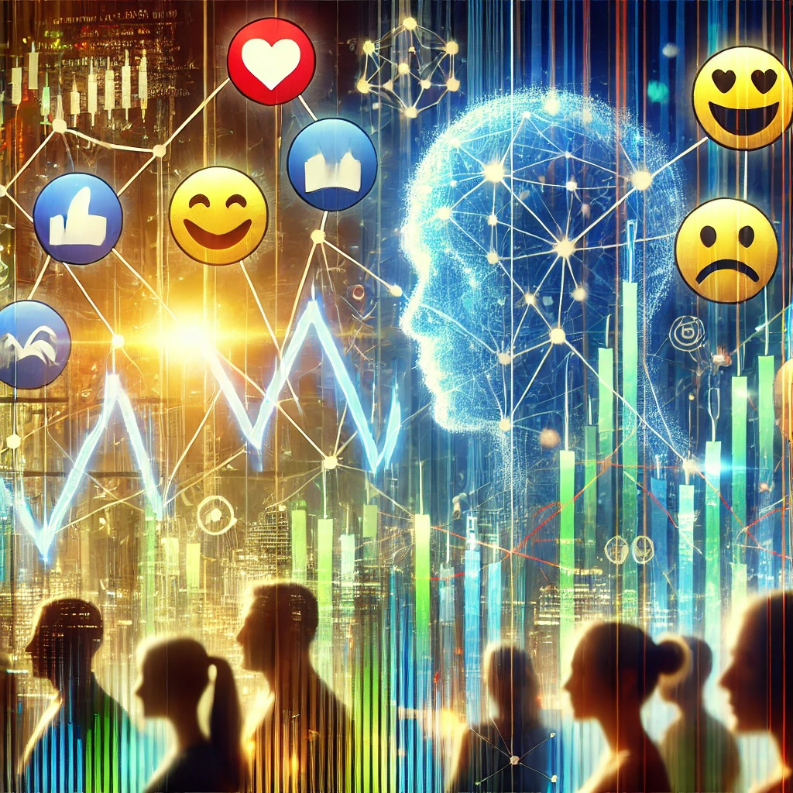
Machine learning (ML) can be a powerful tool for predicting and managing market risks by analyzing vast amounts of data, identifying patterns, and providing insights that help anticipate market movements and mitigate risks. Below are some ways in which machine learning can be applied in this context:
1. Predicting Market Trends and Price Movements
Time Series Forecasting: ML models, such as ARIMA (Auto-Regressive Integrated Moving Average) or deep learning techniques like Long Short-Term Memory (LSTM) networks, can be used to forecast future market trends based on historical price data, trading volumes, and other financial indicators.
Sentiment Analysis: ML algorithms can process social media, news articles, and financial reports to gauge market sentiment. By analyzing this data, ML models can predict how news events (such as earnings reports, geopolitical events, or central bank announcements) might influence market movements.
Regression Models: Linear or non-linear regression models can predict asset prices or market movements by identifying relationships between market variables (e.g., interest rates, economic indicators) and asset returns.
2. Risk Classification and Portfolio Optimization
Risk Profiling: Machine learning can be used to develop risk profiles for individual assets or entire portfolios. By analyzing historical returns, volatility, and other risk factors, ML models can classify assets into different risk categories (e.g., high, medium, or low risk).
Portfolio Optimization: ML algorithms such as reinforcement learning can help optimize asset allocation by maximizing returns for a given level of risk. For example, algorithms can continuously learn and adjust portfolios based on changing market conditions and risk appetite.
3. Predicting and Managing Volatility
Volatility Forecasting: ML models can predict market volatility by analyzing historical volatility data and market conditions. Techniques like GARCH (Generalized Autoregressive Conditional Heteroskedasticity) models, combined with ML algorithms, can improve volatility forecasts, which are critical for managing financial risks.
Option Pricing: ML models, including neural networks, can be used to predict option prices by considering a wide range of factors that affect market conditions (e.g., asset price, time to expiration, volatility). These predictions help traders hedge risks associated with price fluctuations.
4. Anomaly Detection
Fraud Detection: ML algorithms can be used to identify unusual trading patterns that might indicate fraud or market manipulation. Anomalies such as sudden spikes in trading volume or price movements can trigger alerts for further investigation.
Operational Risk Management: ML can help identify operational risks by analyzing patterns in trading activities, such as sudden errors in pricing, technology failures, or misalignment with risk management policies. Detecting these anomalies can prevent larger issues from arising.
5. Stress Testing and Scenario Analysis
Stress Testing: ML models can simulate a wide variety of market scenarios (e.g., economic downturns, interest rate hikes, geopolitical crises) to understand how a portfolio or asset would perform under extreme conditions. These simulations help institutions assess their exposure to different types of risks and prepare for potential adverse events.
Scenario Analysis: Machine learning can automate and enhance scenario analysis by simulating different market conditions and assessing how assets or portfolios would behave under those conditions. This helps in planning for extreme but plausible market shocks.
6. Credit Risk Assessment
Credit Scoring Models: Machine learning can be applied to predict the creditworthiness of borrowers by analyzing a range of data beyond traditional credit scoring models, such as transaction history, social media activity, and other non-financial data. This enables more accurate risk assessments and better decision-making regarding lending.
Default Prediction: ML can be used to predict the likelihood of a borrower defaulting by analyzing historical data on borrowers with similar characteristics. Models like decision trees, random forests, and gradient boosting machines are often used for this purpose.
7. Algorithmic Trading for Risk Management
Market Liquidity Analysis: ML models can analyze order books and trading patterns to predict liquidity conditions, helping traders manage risks related to market liquidity.
Risk-adjusted Trading Strategies: ML algorithms can be used to develop dynamic trading strategies that adjust risk levels in real-time based on current market conditions. For example, if a model predicts increased volatility, it can reduce exposure to high-risk assets automatically.
8. Risk-Adjusted Returns Evaluation
Sharpe Ratio Optimization: Machine learning techniques can be used to evaluate risk-adjusted returns, such as the Sharpe ratio, by incorporating a wide range of factors beyond traditional models. This allows investors to better understand the risk-reward trade-off in their portfolios.
Value at Risk (VaR) Prediction: ML can help calculate and predict Value at Risk (VaR), which estimates the potential loss in portfolio value over a given time horizon under normal market conditions. By learning from past market data, ML models can more accurately estimate potential losses.
9. Market Sentiment and Behavioral Analytics
Behavioral Risk: ML can detect patterns in investor behavior that might indicate psychological biases or irrational actions, such as herd behavior, panic selling, or overconfidence. By analyzing these patterns, firms can manage behavioral risks and mitigate the impact of investor psychology on the market.
Sentiment-Driven Risk: By integrating sentiment analysis into market risk models, ML can help assess the impact of market moods, social sentiment, or investor confidence on price fluctuations and potential risk factors.
10. Hedging and Derivatives Management
Hedging Strategies: ML can optimize hedging strategies by predicting price movements and volatility. This enables better management of exposure to market risks, ensuring that the hedges are effective under different market conditions.
Dynamic Hedging: Machine learning can adjust hedging strategies dynamically based on real-time market data, helping traders and investors reduce their exposure to fluctuations in asset prices.
Conclusion
Machine learning offers robust tools to predict and manage market risks by identifying trends, forecasting volatility, detecting anomalies, optimizing portfolios, and assessing various forms of risk (credit, operational, market). By applying machine learning techniques, financial institutions can enhance their ability to anticipate potential risks, optimize risk-adjusted returns, and automate risk management processes, ultimately contributing to more stable financial systems and better-informed decision-making. However, it's important to combine ML with human expertise, regulatory oversight, and ethical considerations to ensure responsible use.
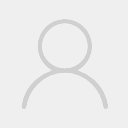