How can XAI ensure fairness and eliminate biases in AI systems?
How can XAI ensure fairness and eliminate biases in AI systems?
by Maximilian 10:19am Feb 01, 2025
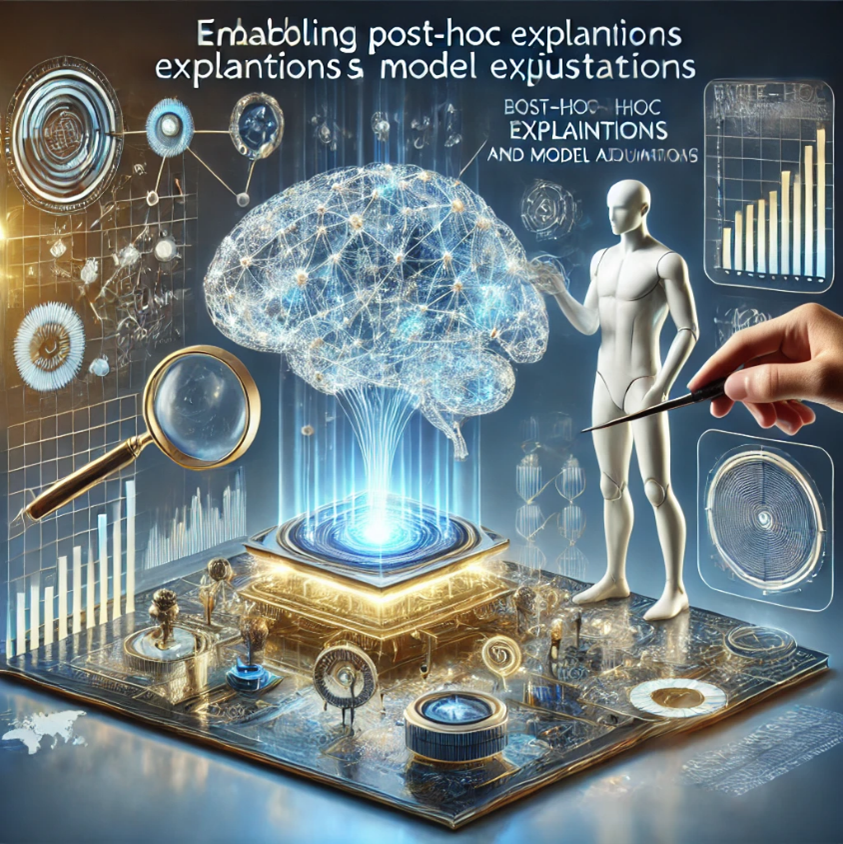
Explainable AI (XAI) can play a crucial role in ensuring fairness and eliminating biases in AI systems by making machine learning models more transparent and interpretable. By making AI decision-making processes clearer to humans, XAI can help identify and address issues related to fairness and bias that might otherwise go unnoticed. Here’s how XAI can contribute to these goals:
1. Improving Transparency in AI Decision-Making
Understanding Model Behavior: XAI tools can help reveal how an AI system arrives at its decisions by providing interpretable explanations of the model's inner workings. For example, decision trees, feature importance, or counterfactual explanations can explain which features influence a model’s prediction and how.
Bias Detection: By understanding how a model makes decisions, data scientists and stakeholders can inspect whether certain demographic groups (e.g., gender, race, age) are disproportionately influencing the outcomes.Transparent models allow for a deeper investigation into whether decisions are being made based on biased patterns in the data or the model's design.
Example: If a loan approval model consistently rejects applicants from a particular ethnic group, XAI tools can reveal if certain features related to ethnicity or zip codes are being weighted too heavily. This transparency allows for corrective measures to be taken.
2. Enabling Fairness Audits
Bias Identification: XAI facilitates fairness audits by providing human-readable explanations of decisions. These audits can be used to check if the AI system is favoring certain groups over others. Fairness audits rely on the ability to examine the decision process for indicators of bias, such as unequal treatment based on protected attributes like race or gender.
Example: In hiring algorithms, XAI can help evaluate whether the model is systematically favoring one gender over another, based on the explanations for decisions it makes (e.g., weighting certain qualifications that may disadvantage one gender over others).
Fairness Metrics: XAI can support the calculation of fairness metrics, such as demographic parity, equalized odds, or disparate impact, by allowing the system to explain how decisions affect different subgroups. These metrics can be tracked and adjusted to ensure fairness in outcomes.
3. Detecting and Mitigating Data Bias
Bias in Training Data: Many AI models inherit biases present in the training data. XAI can help detect these biases by providing insights into how different features are influencing predictions. If certain features (e.g., zip code, income level) are correlated with biased outcomes (e.g., discrimination against certain racial or socioeconomic groups), these biases can be identified and corrected.
Example: In a predictive policing system, if the model is using historical crime data that reflects biased policing practices, XAI can show how these biased data points are influencing the model’s predictions, enabling the identification and removal of unfair biases from the data.
Preprocessing and Rebalancing: Once biases in the data are identified, XAI can guide the development of methods to balance the data. This could involve re-weighting or resampling the data, removing biased features, or using techniques like adversarial debiasing to reduce bias during model training.
4. Incorporating Ethical Decision-Making
Incorporating Stakeholder Perspectives: XAI can enable stakeholders, including marginalized groups or experts in ethics, to understand AI decisions and provide feedback. This can guide the creation of AI systems that reflect diverse ethical standards and avoid harmful biases.
Feedback Loops: XAI enables a feedback loop where the impact of AI decisions on various groups can be observed in real-time. For example, if a facial recognition system disproportionately misidentifies people from certain demographic groups, XAI can help track the source of the issue (e.g., feature selection, dataset imbalance), prompting refinements and updates to the model.
Human Oversight: XAI ensures human oversight by making AI systems understandable. With the ability to interpret AI decisions, humans can better judge whether the AI system is making ethical or fair choices, ensuring accountability.
5. Enabling Post-Hoc Explanations and Model Adjustments
Post-Hoc Explanations: Even if an AI system is not inherently interpretable, XAI techniques can provide post-hoc explanations after the system makes a decision. These explanations can show which features were important in the decision-making process and how they might reflect or exacerbate biases. This allows for corrective actions to be taken even after a decision has been made.
Example: In a criminal justice setting, if a predictive risk model assigns a higher recidivism risk score to individuals from a particular ethnic background, XAI could help explain the factors leading to the decision (e.g., past arrest data, socioeconomic status). These insights can drive efforts to correct the model's fairness by adjusting the model or eliminating harmful data features.
6. Supporting Fair Model Design
Fairness Constraints and Adjustments: XAI can help developers set fairness constraints during the design and training of AI models. For example, XAI techniques can show how changes to the model’s structure or training procedure impact fairness. By iterating on these models, developers can fine-tune them to be both accurate and fair.
Bias Mitigation Algorithms: XAI can assist in applying and monitoring fairness algorithms that aim to mitigate bias during model training. These algorithms may focus on adjusting the data, altering the model’s decision thresholds, or using regularization techniques to ensure that predictions are not biased toward particular groups.
7. Building Trust and Accountability
Stakeholder Trust: By making AI decisions explainable, XAI builds trust with users, especially those who may be directly affected by the system, such as job applicants, patients, or consumers. Users are more likely to accept AI-driven outcomes if they can understand and verify how decisions are being made.
Regulatory Compliance: In many industries, such as finance, healthcare, and employment, organizations are required to ensure that AI systems comply with fairness and anti-discrimination regulations. XAI can provide the transparency and documentation needed to demonstrate compliance with legal and ethical standards.
Conclusion
XAI can significantly contribute to eliminating biases and ensuring fairness in AI systems by providing transparency, enabling audits, and offering tools for bias detection and mitigation. By making AI decisions understandable and interpretable, XAI allows stakeholders to scrutinize and adjust AI systems to be more equitable. Through careful analysis, human oversight, and iterative improvement, XAI helps ensure that AI systems are both accurate and fair, promoting ethical AI deployment across various sectors.
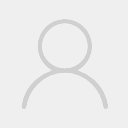