How close are we to creating energy-efficient AI chips for real-time edge computing?
How close are we to creating energy-efficient AI chips for real-time edge computing?
by Maximilian 04:26pm Feb 03, 2025
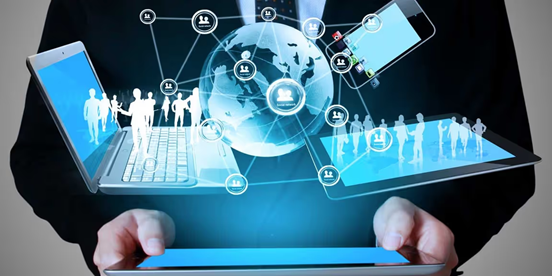
Creating energy-efficient AI chips for real-time edge computing is a rapidly advancing field, and significant progress is being made toward achieving this goal. However, there are still technical challenges to overcome before we can fully realize the potential of these chips. Here's an overview of how close we are to creating energy-efficient AI chips for real-time edge computing:
1. Current Developments and Achievements
Several companies and research institutions have made significant strides in developing energy-efficient AI chips designed for edge computing. These advancements have focused on both hardware design and specialized architectures to optimize power consumption without compromising performance.
Specialized AI Processors: Companies like NVIDIA, Intel, Google, and Qualcomm have developed specialized processors for AI applications. For example, NVIDIA’s Jetson and Google’s Edge TPU are optimized for real-time AI inference on the edge. These chips leverage hardware acceleration (such as tensor processing units, or TPUs) and optimized memory architectures to reduce power consumption during AI tasks, such as object detection, natural language processing, and real-time decision-making.
Low-Power AI Chips: Many AI chips, like those from Ambarella, Graph core, and Cere bras, are designed to run energy-intensive deep learning models with minimal power consumption. These chips leverage technologies like low-voltage operation, custom silicon design, and parallel computing to maximize efficiency. Additionally, FPGA-based solutions (Field-Programmable Gate Arrays) are being increasingly used for energy-efficient, customizable edge computing applications.
Neuromorphic Computing: Neuromorphic chips, like Intel’s Loihi and IBM’s True North, are designed to emulate the brain’s processing capabilities, allowing for extremely low-power operation while performing complex AI tasks in real-time.
2. Key Technological Advances
Some of the main advancements in energy-efficient AI chips include:
Edge AI Accelerators: Chips like Apple’s A14 Bionic chip and Qualcomm’s AI Engine are designed specifically for AI inference on mobile devices and edge applications. These chips integrate multiple cores and accelerators that allow for the fast, low-power execution of machine learning tasks, making them suitable for real-time, on-device AI.
Hardware-Level Optimizations: Many of the new AI chips include optimizations at the hardware level that allow for lower energy consumption. For example, neural processing units (NPUs) and digital signal processors (DSPs) are dedicated hardware accelerators that perform specific AI tasks more efficiently than general-purpose processors.
Energy-Efficient Memory: AI chips for edge computing also incorporate more energy-efficient memory technologies, such as on-chip memory, which reduces the need for power-hungry memory transfers between processors and external storage. 3D memory stacking is another advancement that reduces energy consumption by minimizing latency and power used for data access.
Low-Power AI Algorithms: Software and algorithmic optimizations are also contributing to energy efficiency. Techniques like quantization (reducing the precision of model parameters) and pruning (removing redundant model weights) help reduce the computational load and power requirements of deep learning models, making them more suitable for edge devices.
3. Challenges and Barriers
Despite the progress, several challenges remain in creating fully energy-efficient AI chips for real-time edge computing:
Trade-Offs Between Power and Performance: Achieving both high performance and low power consumption is often a difficult balance. Many energy-efficient chips may reduce power by limiting performance or scalability. For real-time edge computing, where low latency and high throughput are essential, there is still work to be done to ensure that energy efficiency does not significantly compromise performance.
Thermal Management: As AI models grow more complex and demand higher processing power, managing heat dissipation becomes more challenging. Cooling systems and advanced thermal management techniques are essential for keeping AI chips at optimal operating temperatures without excessive power use, but this adds complexity to the design of energy-efficient edge AI systems.
Model Complexity and Size: Many advanced AI models, particularly in deep learning, require significant computational resources, which can consume large amounts of power. Although model compression techniques (like pruning and quantization) have made strides, the size and complexity of models can still be a limiting factor for real-time edge computing, especially when it comes to tasks like image recognition or autonomous driving.
4. Future Outlook
Advances in Custom AI Chips: As more companies invest in custom AI chip design, we can expect to see further breakthroughs in energy-efficient hardware tailored specifically for edge computing. The development of more specialized AI accelerators will help reduce power consumption while maintaining high performance.
AI for Energy Optimization: AI algorithms themselves can be used to optimize hardware design and operations for lower power consumption. For example, AI can be employed to manage the workload of AI chips dynamically, adjusting processing resources based on the complexity of the task, which helps reduce power consumption during less demanding operations.
Quantum Computing and Novel Materials: Long-term, emerging technologies like quantum computing and new materials (e.g., graphene) might provide the breakthrough needed to further enhance the energy efficiency of AI chips for edge computing. These technologies have the potential to revolutionize computing by reducing power consumption for AI workloads.
5. Real-World Applications and Industry Adoption
Autonomous Vehicles: Real-time AI processing for autonomous vehicles requires low-latency and energy-efficient chips to handle tasks like sensor fusion, decision-making, and navigation. Companies like Tesla and Nvidia are already integrating highly energy-efficient AI chips for edge processing in autonomous vehicles.
Wearable Devices and IoT: AI chips for wearable devices (e.g., smartwatches, health monitoring devices) need to be extremely power-efficient to support long battery life while performing continuous tasks like activity recognition or real-time health monitoring. The Apple Watch and similar devices are good examples of edge AI chips optimized for power efficiency.
Smart Cameras and Drones: AI-powered cameras and drones, which perform real-time object detection, face recognition, and environmental analysis, require energy-efficient chips to operate continuously on battery power. Companies like Ambarella are already developing AI chips specifically for video analytics and real-time edge computing in these devices.
Conclusion
We are making significant progress in creating energy-efficient AI chips for real-time edge computing. While current chips already offer a level of energy efficiency and performance suitable for many applications, there are still technical barriers to address, particularly regarding the trade-offs between power consumption and performance, as well as the complexity of AI models. However, ongoing advancements in custom hardware design, optimization algorithms, and emerging technologies suggest that energy-efficient AI chips for edge computing will continue to improve and become more widespread in the near future. Over the next few years, we can expect these chips to become increasingly capable, power-efficient, and adaptable for real-time AI applications on the edge.
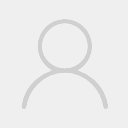