How can machine learning improve warehouse automation and real-time inventory tracking?
How can machine learning improve warehouse automation and real-time inventory tracking?
by Nathaniel 04:09pm Jan 28, 2025
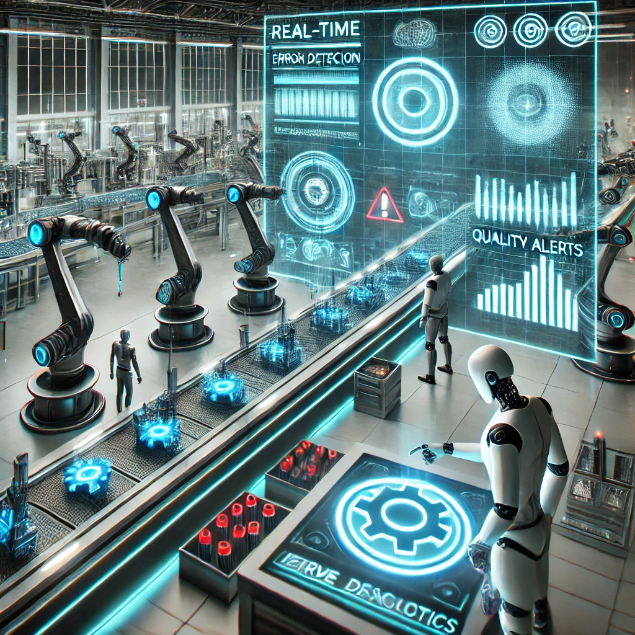
How can machine learning improve warehouse automation and real-time inventory tracking?
Machine learning (ML) can significantly improve warehouse automation and real-time inventory tracking by enhancing operational efficiency, accuracy, and responsiveness. Here are several ways ML can contribute to these areas:
1. Demand Forecasting for Inventory Management
Machine learning algorithms can analyze historical sales data, market trends, and seasonal patterns to accurately predict demand. By providing better demand forecasts, ML helps warehouses maintain optimal stock levels, reducing both overstocking and stockouts. This ensures products are available when needed without tying up excess capital in inventory.
Example:ML models can predict demand spikes during holidays or promotions, helping warehouses prepare in advance with accurate stock levels.
2. Optimized Warehouse Layout and Product Placement
ML can optimize the layout of a warehouse by analyzing the movement of goods, picking frequencies, and order patterns. Based on this data, machine learning algorithms can recommend the most efficient placement for products within the warehouse to reduce the time and energy spent on retrieving items.
Example:Products that are frequently ordered together can be stored closer to each other to minimize the time spent walking or driving through the warehouse to collect orders.
3. Real-time Inventory Tracking with RFID and Sensors
Machine learning can enhance real-time inventory tracking by analyzing data from sensors, barcode readers, and RFID (Radio Frequency Identification) tags. By continuously monitoring the movement of products throughout the warehouse, ML systems can ensure accurate, up-to-date inventory counts and immediately flag discrepancies.
Example:ML can process data from RFID tags to track the real-time location of products within the warehouse. If an item is misplaced or missing, the system will alert managers immediately.
4. Automated Order Picking
Machine learning can be used to optimize the order picking process in warehouses, especially when combined with robotics and automated guided vehicles (AGVs). ML algorithms can analyze past order patterns and optimize routes for picking items, minimizing the travel distance for human workers or robots.
Example:ML-powered robots in a warehouse can dynamically adjust their paths based on order volumes, prioritizing picking high-demand items first and reducing time spent on lower-priority tasks.
5. Predictive Maintenance of Warehouse Equipment
ML can predict when warehouse equipment (such as conveyors, forklifts, or automated picking systems) is likely to need maintenance, based on data collected from sensors. Predictive maintenance minimizes downtime, improves equipment lifespan, and reduces operational costs.
Example:By analyzing historical sensor data, machine learning models can predict when a conveyor belt is likely to malfunction, allowing for preventive maintenance before a breakdown occurs.
6. Supply Chain Optimization
ML can improve the efficiency of supply chain processes that feed into the warehouse, such as optimizing transportation routes and managing supplier lead times. By analyzing various data points, machine learning helps ensure that the warehouse is stocked with the right products at the right time, reducing waiting times and unnecessary storage.
Example: Machine learning models can predict delays in supplier shipments or transportation disruptions, allowing warehouses to adjust their inventory levels and workflows proactively.
7. Automated Sorting and Classification
Machine learning can be used to automate the sorting of goods in a warehouse. ML algorithms can classify products based on various characteristics, such as size, weight, or type, and direct them to the appropriate storage areas or shipping zones.
Example:Vision-based ML systems can scan packages and automatically direct them to the correct storage location or shipping lane, improving sorting speed and accuracy.
8. Intelligent Replenishment Systems
Machine learning can optimize replenishment processes by predicting when stock levels will run low based on real-time sales, order data, and inventory levels. This ensures that products are automatically reordered when they reach a certain threshold, preventing stockouts and ensuring inventory is always at optimal levels.
Example:A warehouse management system powered by ML could automatically order products from suppliers when stock levels reach a predefined limit,preventing unnecessary delays or overstocking.
9. Real-time Error Detection and Quality Control
Machine learning algorithms can be trained to identify errors in real time, such as mispicked items, misplaced products, or incorrect order fulfillment. By analyzing data from cameras, sensors, or even workers’ actions, ML systems can quickly spot mistakes and alert warehouse personnel to correct them.
Example:ML-powered vision systems can monitor packing stations for incorrect labeling or product placement, ensuring that orders are fulfilled accurately.
10. Dynamic Workforce Scheduling
Machine learning can help optimize workforce management by predicting staffing needs based on historical data, seasonal trends, and real-time order volume. This allows warehouses to optimize labor allocation, ensuring that enough workers are available during peak periods without overstaffing during quieter times.
Example:ML models can analyze data from previous months or years to predict when additional staff will be needed, improving efficiency while reducing labor costs.
11. Enhanced Customer Experience through Faster Fulfillment
Machine learning models can reduce order fulfillment time by improving picking, packing, and shipping processes. With better predictions of customer orders and more efficient warehouse operations, ML helps ensure faster shipping and more accurate deliveries, enhancing customer satisfaction.
Example:ML-powered systems can predict which items in a warehouse will be most in demand at a given time and prepare them for quick picking and packing,leading to faster dispatch and shorter delivery times.
12. Warehouse Robotics and Automation Integration
Machine learning algorithms enable warehouse robots and automation systems to learn from experience and improve their efficiency over time. By analyzing data from their environment, robots can adapt their movements, optimize paths, and learn how to handle a variety of tasks, such as picking, packing, or sorting.
Example:ML-driven robots can adapt to new environments, learning how to navigate changes in the warehouse layout or handle new types of products without requiring extensive reprogramming.
Conclusion:
Machine learning is revolutionizing warehouse automation and real-time inventory tracking by enabling smarter decision-making, improving efficiency, and reducing errors. Through demand forecasting, route optimization, predictive maintenance, automated sorting, and dynamic workforce scheduling, ML enhances warehouse operations while also reducing costs and improving customer satisfaction. As these technologies continue to evolve, the role of ML in warehouse operations will only become more significant, driving greater automation and efficiency across the supply chain.
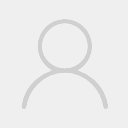