How do surveillance AI systems contribute to societal inequalities and biases?
How do surveillance AI systems contribute to societal inequalities and biases?
by Nathaniel 04:18pm Jan 30, 2025
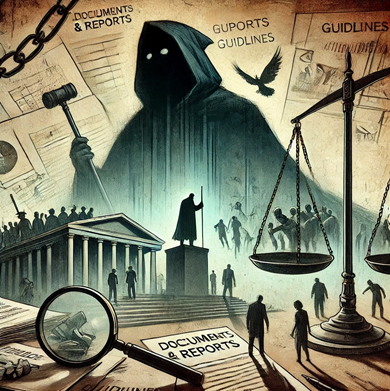
How do surveillance AI systems contribute to societal inequalities and biases?
Surveillance AI systems, while promising enhanced security and efficiency, can inadvertently contribute to societal inequalities and biases. These systems, such as facial recognition or predictive policing, are often built using algorithms that may reflect or exacerbate existing societal disparities. Here are several key ways in which these AI systems contribute to inequalities and biases:
1. Racial and Ethnic Bias in Facial Recognition
Facial recognition technology is one of the most widely used surveillance AI systems, but studies have shown that these systems tend to exhibit significant racial and ethnic biases.
Inaccurate identification of people of color: Many facial recognition algorithms are less accurate at identifying people of color, particularly Black, Asian, and Hispanic individuals, compared to white individuals. This is due to underrepresentation of these groups in training data, which skews the accuracy of the systems. As a result, people of color are more likely to be misidentified, which can lead to false arrests or wrongful surveillance.
Example:Research by the National Institute of Standards and Technology (NIST) found that some commercial facial recognition systems have a much higher error rate for identifying Black faces compared to white faces. This discrepancy can lead to unjust profiling and over-surveillance of minority communities.
Over-surveillance of marginalized communities: Facial recognition and other surveillance systems are often deployed disproportionately in areas with higher populations of marginalized groups. This can lead to increased surveillance and a higher likelihood of people from these communities being monitored, targeted, or criminalized based on inaccurate data.
2. Bias in Predictive Policing
Predictive policing uses AI algorithms to analyze historical crime data and predict where future crimes are likely to occur. However, the data used to train these systems can reflect and perpetuate biases that already exist in the criminal justice system.
Reinforcing historical inequalities: If a predictive policing system is trained on historical arrest or crime data, it may learn patterns that reflect systemic biases within law enforcement, such as racial profiling or over-policing in specific communities. As a result, the algorithm may disproportionately predict crime in historically over-policed neighborhoods, which often have a higher population of Black, Hispanic, and low-income individuals.
Example:Systems like PredPol have faced criticism for reinforcing racial disparities. The algorithms can perpetuate cycles of surveillance and policing in already marginalized communities, where high arrest rates may be driven by systemic bias rather than higher crime rates.
Disproportionate targeting: Predictive policing systems can direct more police resources toward specific communities based on biased data. This can lead to more frequent stop-and-frisks, arrests, and surveillance in certain neighborhoods, further criminalizing individuals based on biased predictions rather than objective risk factors.
3. Bias in Algorithmic Decision-Making
AI systems used in surveillance may also be used to assist in decisions beyond security, such as job hiring, loan approvals, or housing decisions, all of which can be influenced by biased data.
Discriminatory outcomes: Surveillance data, such as behavioral or demographic information collected from social media or other platforms, can be used to influence automated decision-making systems. If these algorithms are trained on biased data such as racial or gender disparities in hiring practices, for instance this can lead to discriminatory outcomes that disproportionately affect marginalized groups.
Example:In predictive hiring tools, facial recognition, or voice analysis used by some companies, algorithms have been found to discriminate against women or people of color because they are trained on biased datasets that reflect the underrepresentation of these groups in the workforce.
4. Socioeconomic Bias
Surveillance AI systems can also reinforce biases related to socioeconomic status, particularly in how AI interprets and acts on data from economically disadvantaged communities.
Targeting impoverished communities: AI systems that analyze data from urban areas may focus more on neighborhoods with higher poverty rates, leading to over-policing or greater surveillance in these areas. These systems may interpret patterns in socioeconomic behaviors (e.g., high rates of arrests for minor offenses or lower levels of access to legal resources) as indicators of increased crime, which can perpetuate a cycle of monitoring and criminalization in poorer communities.
Example: Algorithms used in predictive policing may incorrectly assume that high-crime areas are more prone to future offenses, overlooking the broader social and economic factors contributing to those conditions. This can lead to greater surveillance and police presence in economically disadvantaged neighborhoods.
Social credit systems:In some countries, AI-based systems are used to track individuals’ behavior, leading to "social credit scores" that can affect their access to services, loans, or even job opportunities. These systems can disproportionately penalize individuals from lower-income or marginalized backgrounds based on their actions or social behavior, even when such actions are influenced by systemic inequalities.
5. Privacy Violations Disproportionately Affecting Vulnerable Groups
Surveillance AI systems often raise privacy concerns, particularly when they are used to monitor individuals in public spaces or collect personal data without informed consent. Vulnerable or marginalized groups may face greater risks of privacy violations.
Lack of consent and control: Many surveillance systems collect data without the explicit consent of individuals being monitored, which can disproportionately impact marginalized groups. For instance, Black and Hispanic communities may be more likely to be subject to facial recognition or biometric monitoring without being adequately informed of how their data is being used.
Chilling effects on freedom: The presence of surveillance AI, especially in areas where people are already vulnerable, can have a chilling effect on freedom of expression and association. Individuals may be less likely to participate in protests, social movements, or political activities if they fear that they are being monitored, particularly in marginalized communities where activism is more likely to be criminalized.
6. Lack of Accountability and Oversight
The lack of transparency and accountability in how surveillance AI systems are deployed exacerbates biases and societal inequalities.
Opaque algorithms:Many AI systems are not transparent, meaning that the public and even the individuals being surveilled may not understand how decisions are being made or how data is being used. This lack of transparency can make it difficult to identify and correct biases, leading to a continued cycle of inequality.
Example:If predictive policing systems or facial recognition software are used without public oversight or external audits, there is a risk that these systems may operate in ways that disproportionately affect marginalized groups without any accountability for the consequences.
Lack of redress mechanisms: In the event of biased surveillance or wrongful identification, there is often a lack of effective mechanisms for individuals to challenge or correct the errors. Marginalized communities, who may already be disadvantaged by the system, are particularly vulnerable in such cases.
7. Exacerbating Systemic Racism and Inequality
Surveillance AI systems, particularly when used in law enforcement or public security, can reinforce existing systemic racism and inequality.
Entrenching discriminatory systems: AI surveillance systems can become tools of systemic oppression if they are deployed in ways that disproportionately target racial or ethnic minorities, low-income individuals, or other marginalized groups. When surveillance tools are primarily used in high-poverty or predominantly minority communities, they can perpetuate discriminatory patterns of policing and criminalization.
Example: The over-policing of Black and Latino communities, already a significant issue in many parts of the world, can be compounded by AI-based surveillance systems that prioritize these neighborhoods for surveillance or law enforcement attention, creating a cycle of bias that reinforces racial inequalities.
Conclusion
Surveillance AI systems, if not carefully designed and regulated, have the potential to exacerbate societal inequalities and biases. These systems can reinforce existing racial, ethnic, socioeconomic, and gender disparities by perpetuating inaccurate identification, over-policing, and discriminatory decision-making. To mitigate these risks, it is essential to ensure transparency, implement bias audits, develop inclusive datasets, establish strong accountability mechanisms, and protect privacy rights. Without these safeguards, AI surveillance could worsen existing societal divides, disproportionately impacting marginalized and vulnerable communities.
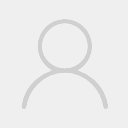